Can computers make better plant-based foods? July/August 2021
By Rebecca Guenard
In This Section
- Microalgae’s impact on human and animal nutrition, April 2024
- Finding purpose and profit from waste, March 2024
- A low-cost, effective green extraction method, February 2024
- Establishing specialized nutrition in China, January 2024
- The disparity between protein sources and their nutritional value, November/December 2023
- Visualizing complex, multiphase food using confocal Raman microscopy, October 2023
- A more sustainable future begins with collaboration and data, September 2023
- Brain diets, July/August 2023
- Green coffee beans meet green tech, June 2023
- Fatty acid intake and inflammation, May 2023
- The Wild West of edible oils, April 2023
- The arc of preservation bends toward nature, March 2023
- Cloaking a cure: Lipid capsules save the world, February 2023
- From the farmers market to store shelves, January 2023
- Dogma vs. data: Rethinking linoleic acid, November/December 2022
- Improving food packaging, October 2022
- Proteins for meat alternatives: new competition for soy and wheat, September 2022
- Fish heads, fish heads: For biosurfactant production July/August 2022
- Mimicking mother’s milk June 2022
- Three paths forward for sustainable palm oil May 2022
- The continued struggle over biofuel feedstocks March 2022
- A new kind of plant breeding February 2022
- The new bio-based surfactant feedstock January 2022
- Lipid role in the immune system November/December 2021
- New essential dietary lipids? October 2021
- Gut Instincts September 2021
- Can computers make better plant-based foods? July/August 2021
- Poisson from a petri dish June 2021
- The latest additions to eco-friendly cleaning May 2021
- Preserving emulsions with plant-based antioxidants April 2021
- Developments in green surfactants for enhanced oil recovery March 2021
- Alternative base oils: a perspective March 2021
- The COVID-19 pandemic, one year later March 2021
- The green machine: commercializing microalgae products February 2021
- Bio-based (edible) oils: feedstock for lubricants of the future January 2021
- The latest on liposomes January 2021
- Fatty acids and athletic performance November/December 2020
- Where are lubricants headed November/December 2020
- New developments in vegetable oil materials science October 2020
- Agriculture at risk: preparing the oilseed industry for a warmer world September 2020
- Science highlights from a cancelled 2020 AM&E July/August 2020
- Managing your career in times of change June 2020
- Lipidomics comes of age May 2020
- Minimally processed oils April 2020
- The high-throughput frontier March 2020
- Nurturing innovation: how AOCS industries are fostering progress February 2020
- The trouble with studying omega-3s and the brain January 2020
- Understanding pulse anti-nutrients January 2020
- Digitizing manufacturing: how companies are using data to improve production November/December 2019
- Weaving together genetics, epigenetics, and the microbiome to optimize human nutrition October 2019
- Taking the cream out of ice cream September 2019
- Science highlights from St. Louis July/August 2019
- Biotechnology conquers consumer goods June 2019
- Cool characterization methods and where to find them May 2019
- Fermentation, the new protein supply chain April 2019
- Oleogels for drug delivery March 2019
- The complexity of clean-label cosmetics February 2019
- Rethinking plastic packaging January 2019
- Trends in synthetic and natural antioxidants for shelf life extension of meat and meat products November/December 2018
- The icing on the cake October 2018
- Enhancing oxidative stability and shelf life of frying oils with antioxidants September 2018
- Under arrest: investigating factors that govern partial coalescence July/August 2018
- Unconventional Oils June 2018
- Beauty from within May 2018
- Pulses rising April 2018
- Lessons learned from Hurricane Harvey March 2018
- Clean meat February 2018
- What makes your shortening suitable for fancy croissants, puff and Danish pastry? January 2018
- Strategic role of peanuts in sustainable global food security November/December 2017
- Science beyond borders: international student exchange October 2017
- Clean label: the next generation September 2017
- Science snapshots from Orlando July/August 2017
- Five new AOCS methods June 2017
- The whys and wherefores of life-cycle assessment May 2017
- China’s evolving edible oils industry April 2017
- The mysterious case of the arsenolipids March 2017
- Red palm oil February 2017
- The Highs and Lows of Cannabis Testing October 2016
- Chia: Superfood or superfad? January 2017
- Generational training divide November/December 2016
- Storage stability of roasted nuts and stabilization strategy using natural antioxidants September 2016
- Good vibrations: online and at-line monitoring of edible oils with vibrational spectroscopy July/August 2016
- Benchtop NMR spectroscopy for meat authentication June 2016
- Coconut oil boom May 2016
- Sink or swim: fish oil supplements and human health April 2016
- Pulsed electric field: groundbreaking technology for improving olive oil extraction March 2016
- Prescribing dietary fat: therapeutic uses of ketogenic diets February 2016
- Organogels of vegetable oil with plant wax January 2016
- The power of peptides November/December 2015
- Separation anxiety: membrane cleaning in the 21st century October 2015
- Using direct solid phase extraction to analyze persistent organic pollutants in oily food samples September 2015
- Big fat controversy: changing opinions about saturated fats June 2015
- Use of spent bleaching earth for economic and environmental benefit May 2015
- An introduction to cosmetic technology April 2015
- Food texture and nutrition: the changing roles of hydrocolloids and food fibers March 2015
- Scientists rank thousands of substances according to potential exposure level March 2015
- Clean smell does not always equal clean air February 2015
- Biotechnology: Using living systems to solve problems February 2015
- Flush to fuel January 2015
- 1970s fish oil study criticized January 2015
- Developing a high-performance, low-streak degreaser November/December 2014
- Detection, monitoring, and deleterious health effects of lipid oxidation November/December 2014
- Modified protein mimics taste and texture of fat October 2014
- Development of the first efficient membrane separations of cis fatty acids October 2014
- Regulatory updates on FSMA and combustible dust September 2014
- How enzymes are transforming manufacturing September 2014
- Two advances in biodiesel technology July/August 2014
- 2014 AOCS Annual Meeting & Expo July 2014
- Peanut genome sequenced June 2014
- A customized approach to frying oil June 2014
- Omics reveals subtle changes in carbon flux that lead to increased oil biosynthesis in oil palm May 2014
- Cannabis testing: a review of the current landscape May 2014
- Industrial hemp gaining traction April 2014
- Emulsions: making oil and water mix April 2014
- Lipid co-oxidation of proteins: One size does not fit all March 2014
- FSMA marches on March 2014
- Disruptive technology? Walmart’s “green” product line may signal a big change February 2014
- Pathways to novel chemicals February 2014
- Specialty lipids in pet nutrition January 2014
- EFSA releases preliminary report on occurrence of 3-MCPD in food January 2014
- Seven new biobased surfactant technologies November/December 2013
- Do oil color scales make you see red . . . or yellow? November/December 2013
- Shortage leads to green route to olefins October 2013
- Sesamol: a natural antioxidant for frying oil September 2013
- FSMA update September 2013
- Patent rights and biotech seeds July August 2013
- The other vitamin E July 2013
- Frac fever heats up June 2013
- Fat fight: Catch-22 for Western oleochemicals? June 2013
- Health and Nutrition News April 2013
- FDA asks for fees from industry to fund FSMA June 2013
- What does it take to start a biodiesel industry? April 2013
- What’s in a Claim? Would a Food Not Labeled “Natural” Taste as Sweet? March 2013
- Regulatory overview March 2013
- The preservative wars February 2013
- Plants producing DHA February 2013
- Swift response to paper on feeding GMO corn, glyphosate January 2013
- AOCS: supporting international standards January 2013
- TSCA and the regulation of renewable chemicals July August 2013
- trans Fatty acid content of foods in China January 2013
- A novel green catalytic process for biodiesel production from Jatropha November/December 2012
- The America Invents Act: Groundbreaking US patent law changes are here November/December 2012
- “Super Phos” esters: the key to higher-performance products November/December 2012
- Advances in field-portable mass spectrometers for on-site analytics October 2012
- EFSA sets upper intake level for LC-PUFA October 2012
- Malaysia: economic transformation advances oil palm industry September 2012
- High-oleic canola oils and their food applications September 2012
- Using enzymes to prepare biobased surfactants July/August 2012
- Oilseeds: at the center of food, water, and energy security July/August 2012
- Health & Nutrition News June 2012
- Hydrocolloids get personal June 2012
- The secrets of Belgian chocolate May 2012
- Plants “remember” drought, adapt May 2012
- The power of mass spectrometry in the detection of fraud April 2012
- Oil in biomass: a step-change for bioenergy production? April 2012
- The Future of LAB March 2012
- World supplies of rapeseed and canola likely to remain tight in the 2012/13 season March 2012
- Methods for differentiating recycled cooking oil needed in China February 2012
- Supercritical fluid-based extraction/processing: then and now February 2012
- Singapore: the place to be in 2012 February 2012
- The Food Safety Modernization Act and its relevance to the oilseed industry February 2012
- Oilseeds in Australia January 2012
- Hydrogen peroxide in home-care formulations November 2011
- A new generation of renewable fuels is on the horizon November 2011
- Omega-3 fatty acids: $13 billion global market October 2011
- Soy and breast cancer October 2011
- EU approves food labeling rules September 2011
- IOM panel recommends tripling vitamin D intake: Panel’s conservative approach receives criticism September 2011
- Self-assembly of lyotropic liquid crystals: from fundamentals to applications August 2011
- Sustainability watch July 2011
- Sustainability Watch July 2011
- Are algae really feasible as fuel? June 2011
- The trouble with crystal polymorphism June 2011
- Insect oils: Nutritional and industrial applications May 2011
- Reconstructing formulas April 2011
- US eggs now lower in cholesterol April 2011
- How to control eating behavior--in mice March 2011
- Maybe we don’t know beans March 2011
- Short- and long-term price forecasting for palm and lauric oils February 2011
- New 3-MCPD (glycidol ester) method February 2011
- Regulatory issues associated with the international oils & fats trade January 2011
- Point-counterpoint on UC Davis olive oil report January 2011
- Biomass--The next revolution in surfactants? December 2010
- One person’s response to a high omega-6 diet November 2010
- Crop residues as feedstock for renewable fuels November 2010
- Universal detectors for determination of lipids in biodiesel production October 2010
- New very long chain fatty acid seed oils produced through introduction of strategic genes into Brassica carinata October 2010
- Surfactants based on monounsaturated fatty acids for enhanced oil recovery September 2010
- Questioning the virginity of olive oils September 2010
- Dietary guidelines report released August 2010
- Keeping up with detergent chemistry August 2010
- News from the Expo floor July 2010
- Degumming revisited July 2010
- First high-GLA safflower oil on market June 2010
- AOCS 2.0 debuts June 2010
- Palm fatty acid distillate biodiesel: Next-generation palm biodiesel May 2010
- Palm oil pundit speaks May 2010
- What is unrefined, extra virgin cold-pressed avocado oil? April 2010
- The ultra-low-linolenic soybean market April 2010
- Dealing with the media: A cautionary tale March 2010
- Hempseed oil in a nutshell March 2010
- Carbon management 101: A conversation with Eric Jackson February 2010
- Giants of the Past: Hermann Pardun (1908-2009) February 2010
- Q&A with Bill Christie February 2010
- Update on Jatropha January 2010
- Unique properties of carbon dioxide-expanded lipids January 2010
- The market situation and political framework in Germany for biodiesel and vegetable oil December 2009
- Industrial oil crops-when will they finally deliver on their promise ? December 2009
- Chemically enhanced oil recovery stages a comeback November 2009
- Field-portable mass spectrometers for onsite analytics: What's next? October 2009
- To make biofuels, or not to make biofuels:That is the question. September 2009
- Melamine analysis at the forefront September 2009
- Global oil yields: Have we got it seriously wrong? August 2009
- Omega-3 fatty acid profiling and dietary forensics August 2009
- Oilseeds of the future part 3 July 2009
- The rise and fall of surfactants lore July 2009
- Oilseeds of the future: Part 2 June 2009
- Codex Alimentarius Commission update June 2009
- Raw material sources for the long-chain omega-3 market:Trends and sustainability. Part 3. May 2009
- Oilseeds of the future: Part 1 May 2009
- Chloroesters in foods: An emerging issue April 2009
- Raw material sources for the long-chain omega-3 market: Trends and sustainability. Part 2. April 2009
- Synthetic HDL created March 2009
- Raw material sources for the long-chain omega-3 market:Trends and sustainability. Part 1. March 2009
- A convenient way to increase legume intake February 2009
- Vitamin E’s safety controversy January 2009
- Universal mechanism of aging uncovered? January 2009
- Is it time to reconsider the role of saturated fats in the human diet? April 2022
July/August 2021
- Instead of replacing the protein source in a formulation and then masking the off-flavors of plant-based ingredients, companies are determining what fundamentally makes a product taste good.
- These companies are collecting vast amounts of data on everything from subjective human responses to precise analytical measurements.
- They plan to implement statistical modeling and machine learning to determine how to formulate for great tasting plant-based foods.
Until recently, drug development meant addressing the mechanics of a disease. Cancer treatments, for example, were primarily geared toward slowing the rapid division of tumor cells. With the establishment of high-throughput analysis, researchers began approaching drug discovery differently. They turned to producing large volumes of complex and diverse data sets to identify drug targets within individual genes.
As they gathered more genetic data, researchers improved statistical models to predict the location of genome mutations and worked out how to synthesize molecular remedies to correct those errors. They wrote algorithms to train computers how to make such predictions faster. Then they gathered more data to fine-tune their algorithms. Data science is now a core discipline in pharmaceutical research, and food companies are beginning to adapt this approach to their industry, particularly in solving the problem of off-flavors in plant-based foods (Fig 1).
“There is a rational development process to determine what works and what does not to get us closer to our goal,” says Rick Gerkin, associate professor at the University of Arizona in Tempe. “Our goal is ultimately making something that is appealing to a consumer, is healthy and is made from plants.”
Gerkin collaborates with a Berkeley, California start-up called Climax Foods that is using data science to guide the formulation of plant-based creations for seven of the most popular dairy cheeses. The company is collecting data along every step of their cheese production process and comparing it with the sensory perceptions of tasters who comment on the final product. As their data sets grow, they will use them to build statistical models for the formulation of the ideal plant-based cheese that mimics the taste, texture, and nutrition of a dairy cheese. “There is a list of things that are unavailable to us when making a plant-based, all natural product,” says Gerkin. “Under those constraints we have to find new formulations.”
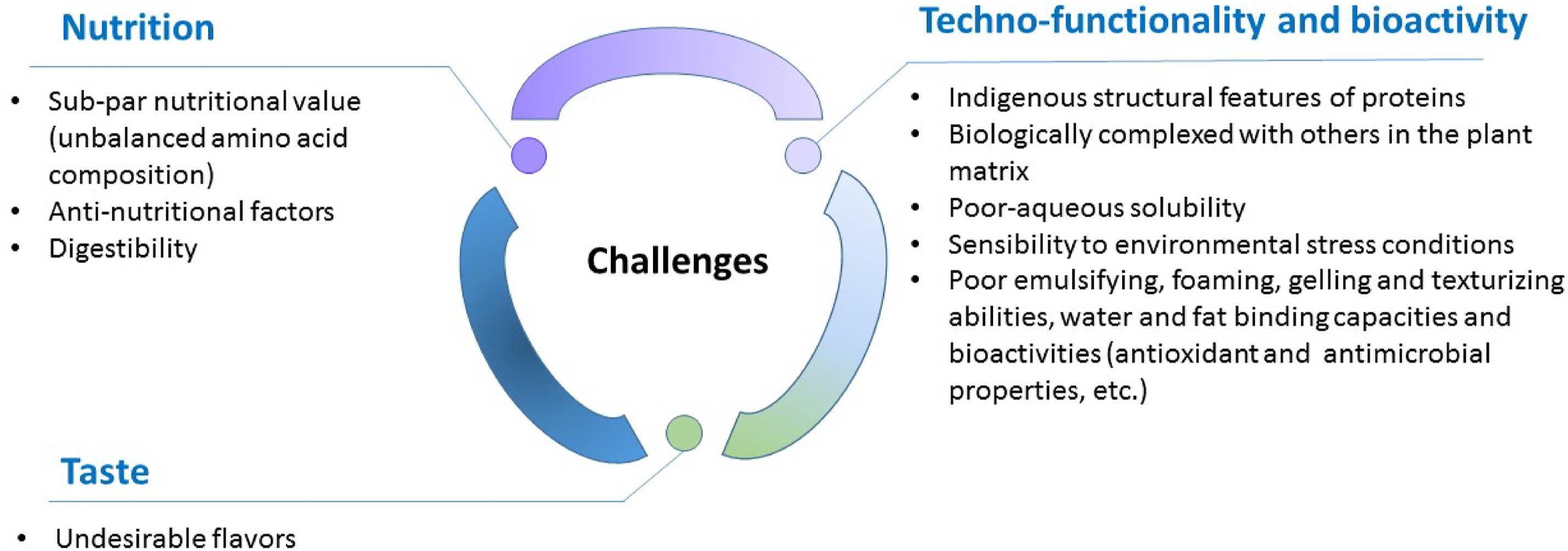
Finding the right protein
Boston-based food ingredient company, Motif Foodworks, is engaged in a similar pursuit. Dilek Uzunalioglu, Motif's head of product applications, says her company is looking for the fundamental factors that make plant-based foods “cravable”. Motif is focused on overcoming the off-flavors and unpleasant textures consumers commonly describe as part of the plant-based food experience. Uzunalioglu says her company is working on a variety of plant-based applications, such as meat and dairy alternatives, but also new forms of plant-based products.
Through collaborations with different experts, Motif is gaining a better understanding of how to select the optimal protein functionality to contribute to specific properties in a finished product. Motif partners with another Boston company called Ginko Bioworks that retrieves genetic information from an extensive library of sequences and uses that information to design new biological products. After screening over 300 proteins in their database, Ginko designs yeast strains that, through fermentation, can make the proteins Motif identifies as potential product ingredients.
This approach helps them select the plant proteins that are best suited for a specific food application and leapfrogs the myriad physical and chemical modifications needed to make some plant proteins more palatable for food applications. “It is really about gathering the insights and then looking for gaps where we can design proteins or any other ingredients to remove the gap,” says Uzunalioglu. “Scientific biology is one of the tools we use, but it is not the only one.” Adding genetic tools to existing analytical techniques, like rheology, helps pinpoint proteins destined to function in food applications while also achieving consumer satisfaction.
Following the drug discovery playbook, Motif is applying high-throughput analysis to characterize a large number of samples at once and screen for the traits that interest them. Automated preparation in multi-well plates followed by simultaneous sample analysis allows pharmaceutical companies to conduct a fast screening of hundreds of potential new product formulations (see Inform, March 2020). Drug developers can calculate and optimize experimental conditions, such as buffers, surfactants, sugars, storage temperature, and mechanical stress, much faster using these methods.
Uzunalioglu says, through a collaboration with researchers at the University of Massachusetts, Motif has developed two-gram samples of protein assays for use in high-throughput measurements. The capability allows them to quickly determine the foaming, gelling, and emulsification properties of any protein they produce through fermentation.
The data gathered from these measured properties can then be associated with the traits that consumers describe in a food, such as moistness and chewiness. “For moistness, we measure water holding capacity and oil holding capacity,” says Uzunalioglu. “For chewiness, we measure water holding capacity and gelling and foaming, things like that.” She says that Motif uses a combination of trained tasters and the general public to gather information on how consumers experience different ingredients.
Motif’s strategy of combining their analytical results with consumer satisfaction is indicative of the reality that, ultimately, human perception decides a product’s success. Formulators know that fragrances and mouthfeel play a crucial role in perception. Many hope to equate human experience with specific molecules, but measuring perception is complicated.
Interpreting sensory perceptions
Unlike with vision, sound, or touch, which researchers can track from an input to a neurological signal in humans, odor perception is harder to pin down. Instead of proceeding directly to the thalamus like other sensory systems, scent signals first travel to brain regions that process emotions and memory. Smell has a profound impact on how we experience food. For instance, those who lost their sense of smell due to the SARS-CoV-2 virus reported that the taste and texture of food had changed (https://tinyurl.com/3x4p3acj).
One way to improve consumer experience with plant-based foods is to identify the source of unpleasantness and avoid ingredients with a similar molecular structure. But that turns out to be a daunting challenge. Vision operates off of four receptors; scent uses 400. Scientists have not yet deciphered the language our olfactory system uses to decode the estimated trillions of different smells the brain understands. However, they have identified enough correlations between physiochemical features and perception to believe the process is structured and not subjective. Therefore, they continue to search for the fundamental molecular features that combine to create a smell.
Earlier this year, University of California, Riverside researchers were able to increase the number of predictable odorant chemicals perceived by the human olfactory system using computational analysis. Genetics, culture, and lived experience all contribute to a sense of smell, but enough similarities in descriptions of smell for the same chemical exist to imply a common physiochemical basis for their perception among humans. The UC researchers set out to train computers to identify the small group of physiochemical traits that were determined in earlier experiments and, using machine learning, see if the computer could then find other traits.
The team used databases from previous experiments that contained odor character profiles—describing smells such as cooked meat, cooked vegetables, or green vegetables— along with the physiochemical description of the compounds. They wrote algorithms to rank chemical features in terms of their contribution to those descriptors. Then they applied machine-learning models to predict the smell humans were most likely to perceive from specific features.
The group reported that using their statistical models, they were able to extend data from what they referred to as “low-throughput, high-cost human studies” and explore new areas of chemical perception. From a library of 440,000 chemicals, the models were able to predict the descriptions of the smells that human participants had given in previous studies. In addition, the researchers introduced 68 million word combinations that describe smells into the model and identified new chemicals that smell like each descriptor (Fig. 2). This chemical discovery aspect of the research could be lucrative to food and ingredient manufacturers. Machine learning may help unveil previously unknown compounds that remind humans of the smell of freshly cooked meat, for example, even for a meatless product.

Managing all the data
At the University of Arizona, Gerkin conducts research similar to the work performed by the UC team (though he uses statistical models to interpret neurological activity in response to odor). He has written that the predictive modeling used by the UC team is a valuable way to find new flavor compounds. His collaboration with Climax Foods could signify that the start-up is interested in making such an attempt. However, when asked, Gerkin said, for now the partnership is focused on “trying to figure out what is the very best way to apply the cutting edge of the academic work into industry.” That means gathering as much data as possible on the fermentation process of cheese.
“You want to connect the variable that you can control in your process to something you can measure—either by a panel of people evaluating the cheese telling you what they like, or by things you can measure quantitatively,” says Gerkin. Like Motif, they are gathering a variety of data from rheology to GCMS and LCMS. He emphasizes the importance of then knowing what to do with all that data to make it valuable in formulations. Data science is most useful, he says, when you can visualize how all the pieces of information fit together, when you know the uncertainty of all your measurements, and when you can effectively program computers to retrieve valuable predictions from everything you have gathered.
Uzunalioglu says Motif is in the process of data collection that will eventually be used to build predictive algorithms. She confirmed that is the end goal, but for now they are collecting as wide a variety of data as possible. Along with biotechnology and tasting panels, Motif is gathering research on psychology and oral processing (see “Improving mouthfeel data”). Uzunalioglu says, incorporating a breadth of information is part of her company’s development philosophy.
During the pandemic, sales of plant-based foods increased due to meat shortages that occurred when outbreaks hit processing plants. Matt Roszell, head of Motif’s marketing communications, says that was an opportunity to gain new customers in the plant-based space, but many did not adopt the products permanently because they did not love their experience. “Plant-based foods are never going to be more sustainable or better for people’s health if they just do not eat them,” he says.
To get to that next level of customer acceptance, food scientists need more options than covering up odd flavors or textures with added sugar and salt to hide the earthiness of plant proteins. And, they want to know if anything other than gums and hydrocolloids can replicate the performance of animal fats.
Uzunalioglu and Roszell say they expect that the arsenal of data and computational power Motif has applied to determining what piques consumers’ taste buds will lead to an indistinguishable eating experience between plant-based foods and native meats within one or two years.
Improving mouthfeel data
Plant-based food manufacturers often want to balance high protein in formulation with low fat and low sugar. Unfortunately, this combination typically leads to a product that consumers describe as chalky or gritty. Over the past decade, researchers have been investigating the components of mouthfeel hoping they might reveal a clue to avoiding unpleasant textures, but a recent review of saliva-protein interaction experiments found more experiments are needed.
The sensory perception and after taste a person experiences when eating is related to saliva’s interaction with the food. The bio-lubricant coats mouth surfaces and assists with food processing. Saliva is mostly water, but contains proteins and ionic compounds that influence our perception of texture, especially when interacting with other proteins.
A team of researchers at the University of Leads, Leads, UK, performed what they believe is the first systematic review of protein-saliva studies confirming that these interactions are dominated by electrostatic charges strongly influenced by pH. However, the findings were exclusively for dairy proteins and, according to the researchers, the literature did not yet contain an analysis of plant protein–saliva interaction to predict mouthfeel perception.
Furthermore, the systematic review indicates that the current body of work on protein-saliva interactions is flawed. The review authors suggest that some of the methodology does not reflect a physiologically relevant saliva-to-protein ratio and is, therefore, an inaccurate simulation. In addition, there was a lack of similarity across studies making comparisons difficult. They suggest that in the future, standardization be applied to protein-saliva experiments to improve research quality and enable comparisons.
As data scientists become confident in the application of predictive models, mouthfeel data is likely one of the components they will be interested in incorporating in their statistics. First, they must be certain that data is relevant and reliable.
About the Author
Rebecca Guenard is the associate editor of Inform at AOCS. She can be contacted at rebecca.guenard@aocs.org
Information
Modification approaches of plant-based proteins to improve their techno-functionality and use in food products, Nasradadi, M.N., et al., Food Hydrocoll 118: 106789, 2021.
Protein–saliva interactions: a systematic review, Brown, F.N., et al., Food Funct. 12: 3324–3351, 2021.
A System-Wide Understanding of the Human Olfactory Percept Chemical Space, Kowalewski, J., B. Huynh, and A. Ray, Chemical Senses 46: 1–13, 2021.
Parsing sage and rosemary in time: the machine learning race to crack olfactory perception, Gerkin, R.C., Chemical Senses 46: 1–5, 2021.